Sampling via Rejection#
Recall that in the lecture, we discussed rejection sampling for two different cases for a \(\text{Beta}(2,2)\) density
Using a uniform proposal density
Using a Gaussian density.
Uniform (box) example had \(M = 1.5\) whereas Gaussian had \(M = 1.3\). Of course the acceptance rates are respectively \(\hat{a}_{\text{box}} = 1/1.5 \approx 0.67\) and \(\hat{a}_{\text{Gauss}} = 1/1.3 \approx 0.76\). We will test this now numerically (by counting the ratio of accepted samples).
Let us first get our basics. We will define the densities and their log-densities (as we do not want to implement the densities due to possible numerical errors).
import numpy as np
import matplotlib.pyplot as plt
rng = np.random.default_rng(52) # random number generator
# compute beta pdf
def beta_pdf(x, a, b):
return 6 * x**(a-1) * (1-x)**(b-1)
def gaussian_function(x, mu, sigma):
return 1/(sigma * np.sqrt(2 * np.pi)) * np.exp( - (x - mu)**2 / (2 * sigma**2))
def log_gaussian_function(x, mu, sigma):
return - np.log(sigma * np.sqrt(2 * np.pi)) - (x - mu)**2 / (2 * sigma**2)
def log_beta_pdf(x, a, b):
return np.log(6) + (a-1) * np.log(x) + (b-1) * np.log(1-x)
Let us now plot the \(\text{Beta}(2,2)\) density and the uniform and Gaussian proposal densities.
# plot beta pdf
xx = np.linspace(0, 1, 1000)
# beta parameters
a = 2
b = 2
m = 0.5 # mean for the Gaussian proposal
v = 0.25 # variance for the Gaussian proposal
M_gauss = 1.3 # for the Gaussian as shown in the lecture
M_box = 1.5 # for the box as shown in the lecture
plt.plot(xx, beta_pdf(xx, a, b), color='k', linewidth=2)
plt.plot(xx, M_gauss * gaussian_function(xx, m, v), color=[0.8, 0.6, 0], linewidth=2)
plt.plot(xx, M_box * np.ones(len(xx)), color=[0.8, 0, 0], linewidth=2)
plt.xlim(0, 1)
plt.ylim(bottom=0)
plt.legend(["$p(x)$", "$M_{\\text{gauss}} q(x)$", "$M_{\\text{box}} q(x)$"])
plt.show()
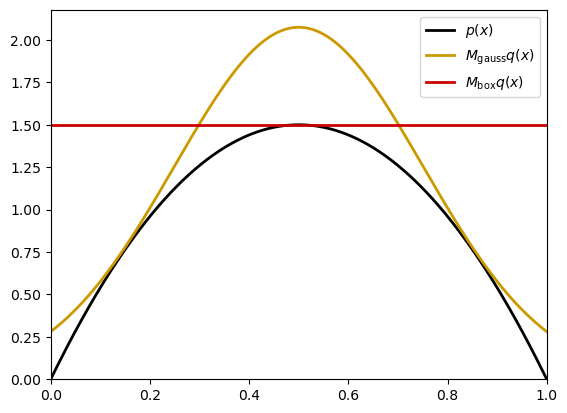
We will now efficiently show a few things
How to implement rejection sampling
Comparison of acceptance rates of two proposal densities as predicted above
Let us write now a sampler function for different proposals.
def beta_rej_sampler_uniform_proposal(N, a, b, M):
# N will be the number of trials but due to rejections, we might not get N samples
# a and b are the parameters of the beta distribution
# M is the maximum of the ratio of the beta pdf and the proposal pdf (hand computed)
acc_samples = np.array([]) # accepted samples
for i in range(N): # writing a for loop for the sake of clarity
x_prop = rng.uniform(0, 1) # sample from the proposal
u = rng.uniform(0, 1) # sample from the uniform distribution
# the following step is u < p / M*q (in the log-domain for stability)
if np.log(u) < log_beta_pdf(x_prop, a, b) - np.log(M):
acc_samples = np.append(acc_samples, x_prop)
acc_rate = len(acc_samples) / N
return acc_samples, acc_rate
def beta_rej_sampler_gaussian_proposal(N, a, b, m, v, M):
# N will be the number of trials but due to rejections, we might not get N samples
# a and b are the parameters of the beta distribution
# m and v are the parameters of the Gaussian proposal
# M is the maximum of the ratio of the beta pdf and the proposal pdf (hand computed)
acc_samples = np.array([]) # accepted samples
for i in range(N): # writing a for loop for the sake of clarity
x_prop = rng.normal(m, v) # sample from the proposal
u = rng.uniform() # sample from the uniform distribution
# the following step is u < p / M*q (in the log-domain for stability)
if np.log(u) < log_beta_pdf(x_prop, a, b) - log_gaussian_function(x_prop, m, v) - np.log(M):
acc_samples = np.append(acc_samples, x_prop)
acc_rate = len(acc_samples) / N
return acc_samples, acc_rate
With these functions at hand, we will now test the acceptance rates.
N = 10000
m = 0.5
v = 0.25
# beta parameters
a = 2
b = 2
M_gauss = 1.3 # for the Gaussian as shown in the lecture
M_box = 1.5 # for the box as shown in the lecture
# uniform proposal
acc_samples_box, acc_rate_box = beta_rej_sampler_uniform_proposal(N, a, b, M_box)
print("Acceptance rate for the box proposal: ", acc_rate_box)
print("Theoretical acceptance rate for the box proposal: ", 1/M_box)
# Gaussian proposal
acc_samples_gauss, acc_rate_gauss = beta_rej_sampler_gaussian_proposal(N, a, b, m, v, M_gauss)
print("Acceptance rate for the Gaussian proposal: ", acc_rate_gauss)
print("Theoretical acceptance rate for the Gaussian proposal: ", 1/M_gauss)
Acceptance rate for the box proposal: 0.67
Theoretical acceptance rate for the box proposal: 0.6666666666666666
Acceptance rate for the Gaussian proposal: 0.7686
Theoretical acceptance rate for the Gaussian proposal: 0.7692307692307692
/var/folders/d8/86whr2t12h70n511w7s7cm_w0000gn/T/ipykernel_14697/2947415207.py:17: RuntimeWarning: invalid value encountered in log
return np.log(6) + (a-1) * np.log(x) + (b-1) * np.log(1-x)
As can be seen from the output, theoretical acceptance rates matches empirical acceptance rates.
We can also plot histograms. For the box proposal, we get the following histogram.
plt.hist(acc_samples_box, bins=50, density=True, color=[0.8, 0, 0], alpha=0.5)
plt.plot(xx, beta_pdf(xx, a, b), color='k', linewidth=2)
plt.show()
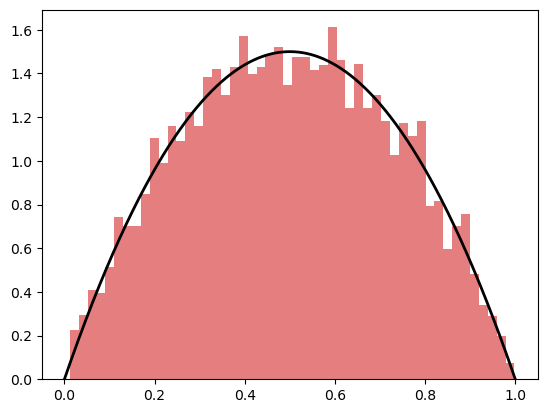
For the Gaussian proposal, we get the following histogram.
plt.hist(acc_samples_gauss, bins=50, density=True, color=[0.8, 0.6, 0], alpha=0.5)
plt.plot(xx, beta_pdf(xx, a, b), color='k', linewidth=2)
plt.show()
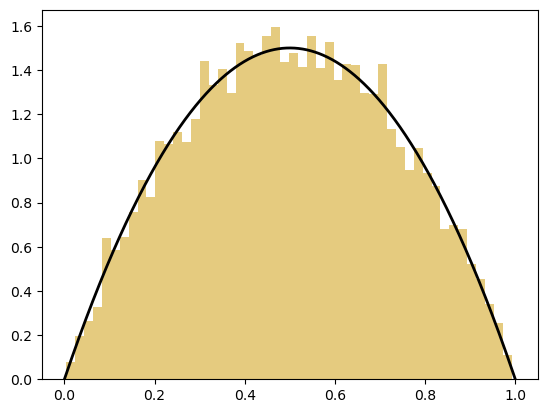